Modelos marginales. Aplicación a datos de panel
Palabras clave:
Modelo marginal, ecuaciones de estimación generalizada, datos tipo panelResumen
El objetivo de este artículo es aplicar el modelo marginal en el análisis de datos tipo panel sobre la situación nutricional de los recién nacidos. Luego de examinar sucintamente los aspectos centrales de los modelos marginales, se revisa brevemente el método de ecuaciones de estimación generalizada (EEG), un método apropiado para la estimación de este tipo de modelos. Con base en un modelo marginal logístico con patrón de correlación intercambiable, se concluye que fumar durante el embarazo y un servicio prenatal inadecuado incrementan significativamente la probabilidad de un nacimiento con bajo peso al nacer.
Descargas
Citas
Agresti, A. (2002). Categorical data análisis (2 ed.). John Wiley & Sons Inc.
Alonso, A., Cano, J., Girón, A., Yep, G. y Sánchez, M. (2005). Peso al nacimiento y tabaquismo familiar. Asociación Española de Pediatria, vol. 3, No. 2, pp. 116-119.
AndreB, H., Golsch, K. and Schmidt, A. (2013). Applied panel data analysis for economic and social surveys. Springer.
Baltagi, B. (2013). Econometric analysis of panel data (5 ed.). John Wiley & Sons.
Banerjee, M. and Frees, E. (1997). Influence diagnostics for linear longitudinal models. Journal of the American Statistical Association vol. 92, pp. 999-1005.
Bickel, P. and Doksum, K. (1977). Mathematical Statistics. Holden-Day.
Bijleveld, C. and Van der Kamp, L.(1998). Longitudinal data analysis: Designs, models and methods. Sage Publications.
Caraballoso, M. (1999). Bajo peso al nacer y tabaquismo. Revista Cubana de Salud Pública vol. 25, No. 1.
Diggle, P., Heagerty, P., Liang, K. and Zeger, S. (2002). Analysis of longitudinal data (2 ed.). Oxford University Press.
Fitzmaurice, G., Laird, N. and Ware, J. (2011). Applied longitudinal análisis (2 ed.). John Wiley & Sons.
Frees, E. (2004). Longitudinal and Panel Data: Analysis and applications for the social sciences. Cambridge University Press.
Gosho, M., Hamada, C. and Yoshimura, I. (2011). Criterion for the selection of a working correlation structure in the generalized stimating equation: Approach for longitudinal balanced data. Communications in Statistics-Theory and Methods No. 40(21), pp. 3839-3856.
Gosho, M. (2014). Criteria to select a working correlation structure for the generalized estimating equations method in SAS. Journal of Statistical Software, vol. 57.
Hardin, J. and Hilbe, J. (2013). Generalized estimating equations (2 ed.). Chapman & Hall.
Hsiao, Cheng. (2003). Analysis of panel data (2 ed.). Cambridge University Press.
Liang, K. and Zeger, S. (1986). Longitudinal data analysis using generalized linear models. Biometrika No. 1, vol. 73, pp. 13-22.
McCullagh, P. and Nelder, J. (1989). Generalized linear models (2 ed.). Chapman & Hall.
Skrondal, A. and Rabe-Hesketh, S. (2004). Generalized latent variable modeling: Multilevel, longitudinal and structural equation models. Chapman & Hall.
Wedderburn, R. (1974). Quasi-likelihood functions, generalized linear models and the gauss-newton method. Biometrika No 61, vol. 3, pp. 439.
Yan, J. and Fine, J. (2004). Estimating equations for association structures. Statistics in Medicine, vol. 23, pp. 859-880.
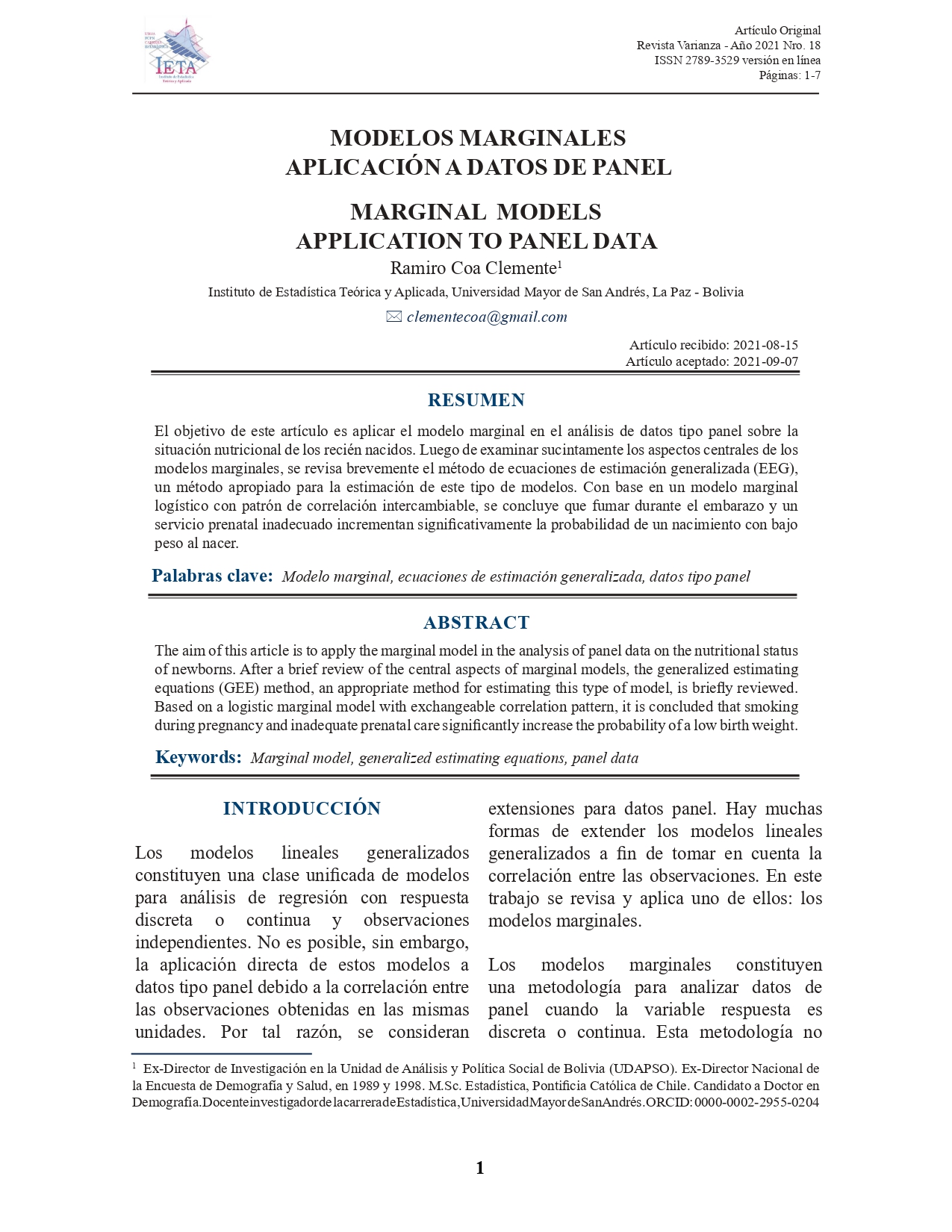